In the last few months, I have learned a lot about modern JavaScript and CSS development with a local toolchain powered by Node 8, Webpack 4, and Babel 7. As part of that, I am doing my second “re-introduction to JavaScript”. I first learned JS in 1998. Then relearned it from scratch in 2008, in the era of “The Good Parts”, Firebug, jQuery, IE6-compatibility, and eventually the then-fledgling Node ecosystem. In that era, I wrote one of the most widely deployed pieces of JavaScript on the web, and maintained a system powered by it.
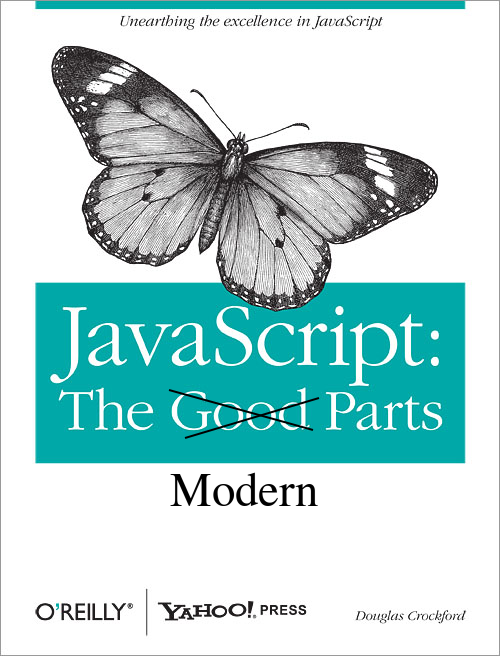
Now I am re-learning it in the era of ECMAScript (ES6 / ES2017), transpilation, formal support for libraries and modularization, and, mobile web performance with things like PWAs, code splitting, and WebWorkers / ServiceWorkers. I am also pleasantly surprised that JS, via the ECMAScript standard and Babel, has evolved into a pretty good programming language, all things considered.
To solidify all this stuff, I am using webpack/babel to build all static assets for a simple Python/Flask web app, which ends up deployed as a multi-hundred-page static site.
One weekend, I ported everything from Flask-Assets
to webpack
, and to play around with ES2017 features, as well as explore the Sass CSS preprocessor and some D3.js examples. And boy, did that send me down a yak shaving rabbit hole. Let’s start from the beginning!
JavaScript in 1998
I first learned JavaScript in 1998. It’s hard to believe that this was 20 years — two decades! — ago. This post will chart the two decades since — covering JavaScript in 1998, 2008, and 2018. The focus of the article will be on “modern” JavaScript, as of my understanding in 2018/2019, and, in particular, what a non-JavaScript programmer should know about how the language — and its associated tooling and runtime — have dramatically evolved. If you’re the kind of programmer who thinks, “I code in Python/Java/Ruby/C/whatever, and thus I have no use for JavaScript and don’t need to know anything about it”, you’re wrong, and I’ll describe why. Incidentally, you were right in 1998, you could get by without it in 2008, and you are dead wrong in 2018.
Further, if you are the kind of programmer who thinks, “JavaScript is a tire fire I’d rather avoid because it lacks basic infrastructure we take for granted in ‘real’ programming languages”, then you are also wrong. I’ll be able to show you how “not taking JavaScript seriously” is the 2018 equivalent of the skeptical 2008-era programmer not taking Python or Ruby seriously. JavaScript is a language that is not only here to stay, but has already — and will continue to — take over the world in several important areas. To be a serious programmer, you’ll have to know JavaScript’s Modern and Good Parts — as well as some other server-side language, like Python, Ruby, Go, Elixir, Clojure, Java, and so on. But, though you can swap one backend language for the other, you can’t avoid JavaScript: it’s pervasive in every kind of web deployment scenario. And, the developer tooling has fully caught up to your expectations.