Frederick Brooks once wrote:
The programmer, like the poet, works only slightly removed from pure thought-stuff. He builds castles in the air, from air, creating by exertion of the imagination.
In his classic essay, “No Silver Bullet”, he also wrote about software’s “essential” complexity:
The complexity of software is an essential property […] Hence, descriptions of a software entity that abstract away its complexity often abstract away its essence. For three centuries, mathematics and the physical sciences made great strides by constructing simplified models of complex phenomena, deriving properties from the models, and verifying those properties by experiment. This paradigm worked because the complexities ignored in the models were not the essential properties of the phenomena. It does not work when the complexities are the essence.
It’s therefore no surprise that once a team develops expertise in a technical domain and starts to evolve a product from that foundation, there is still a huge heap of complexity left over.
This is made worse on bigger problems where more people are involved, thanks to Brooks’s Law. In addition to the software’s essential complexity, you also need to navigate the built-in complexity of human communication, and the associated combinatorial explosion of information pathways on growing teams.
Modern software management practices have thus evolved two distinct skillsets for navigating the problems of team coordination around complex software delivery. These two areas are product management and software engineering management.
Product management centers around areas like market positioning, product strategy, and customer-focused iterative development. The product manager, working closely with the engineers, determines the “what”, aligns the team around the “why”, and acts as a liaison to users and the wider market.
Software engineering management focuses on the practice of coordinating teams of programmers in the delivery of high-quality shipped software, often leveraging programming languages, tools, frameworks, cloud services, and lots of open source technology. Pithily, this is “the how”, as well as the art of making informed engineering tradeoffs, e.g. as relates to time-to-market, performance, quality, and cost.
This post will cover some of my favorite reading materials spanning product management and software engineering management, as disciplines. A preview of some of the titles is here:
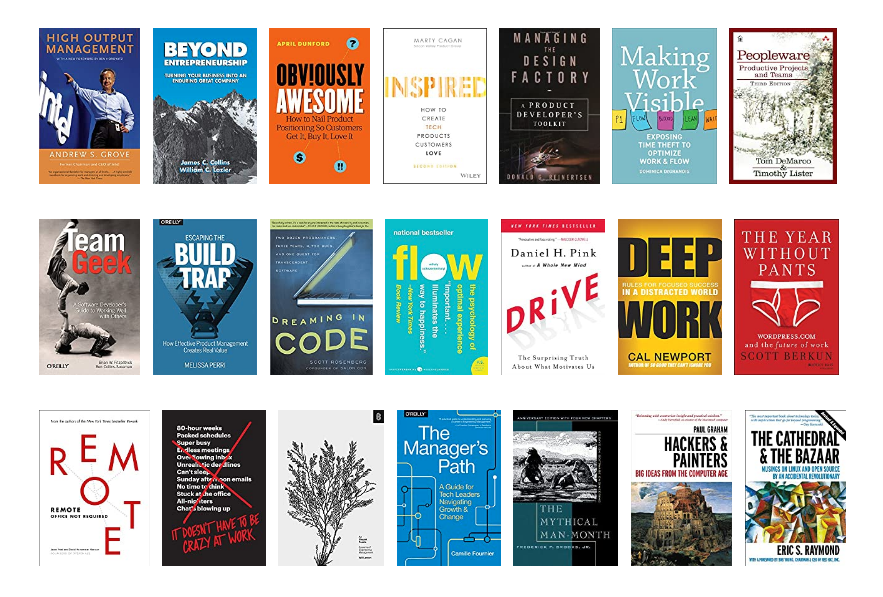
I have curated these resources carefully, and I have recommended them at various times to my own product managers and my own software engineering managers over the years. I hope you find this a useful starting point for your own study.
Continue reading Managing software teams: the definitive reading list